SHORT NEWS
Does this AI think like a human?
Researchers at MIT have developed a method that helps users understand how a machine learning model thinks and compare it to how a human thinks.
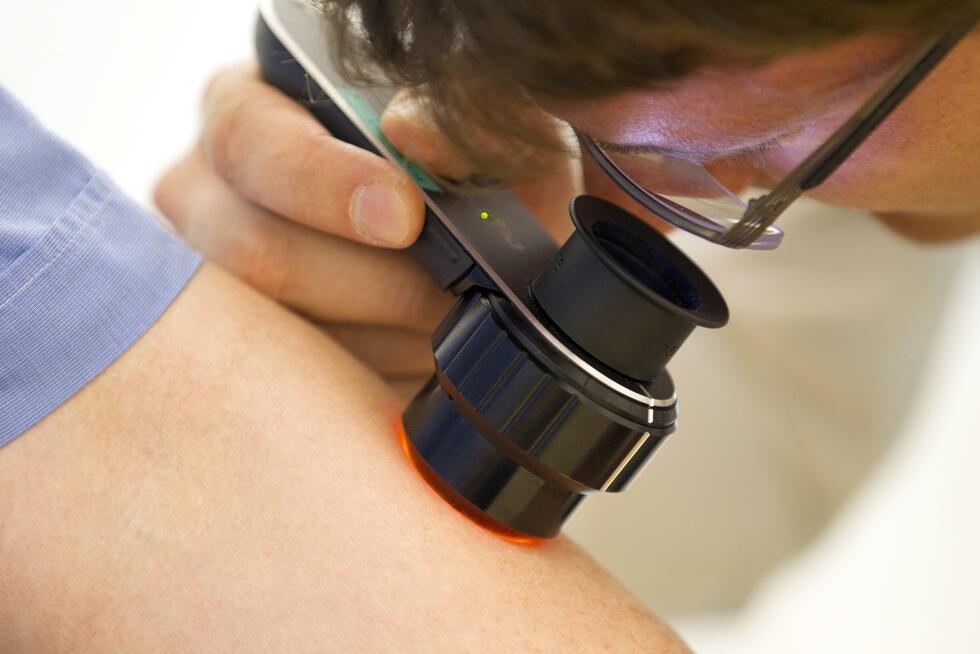
In machine learning, understanding why a model makes certain decisions is often as important as whether those decisions are correct. For example, a machine learning model might correctly predict that a skin lesion is cancerous, but it might also do so based on an unrelated spot in a clinical photograph.
While there are tools to help experts understand a model's reasoning, these methods often only offer insights into one decision at a time - and each must be evaluated manually. Models, however, are typically trained on millions of data inputs, making it nearly impossible for a human to evaluate enough decisions to detect patterns.
Detecting conspicuous behaviour
Researchers at MIT and IBM Research have now developed a method that allows a user to aggregate, sort and score these individual statements to quickly analyse the behaviour of a machine learning model. Their "shared interest" technique involves quantifiable metrics that compare how well a model's reasoning matches that of a human. This would allow a user to easily detect noticeable trends in a model's decision-making - for example, if the model is frequently confused by distracting, irrelevant features such as background objects in photos.