SHORT NEWS
Reducing false alarms in ICUs – thanks to big data
Patient safety in intensive care units could be greatly improved if it were possible to significantly reduce false alarms and predict critical complications such as epileptic seizures. This is where the “ICU Cockpit” project, within the framework of the Swiss National Research Program “Big Data” (NRP 75), comes in: It uses the vast volumes of data generated in intensive care units to develop procedures for early warning systems and therapeutic recommendations.
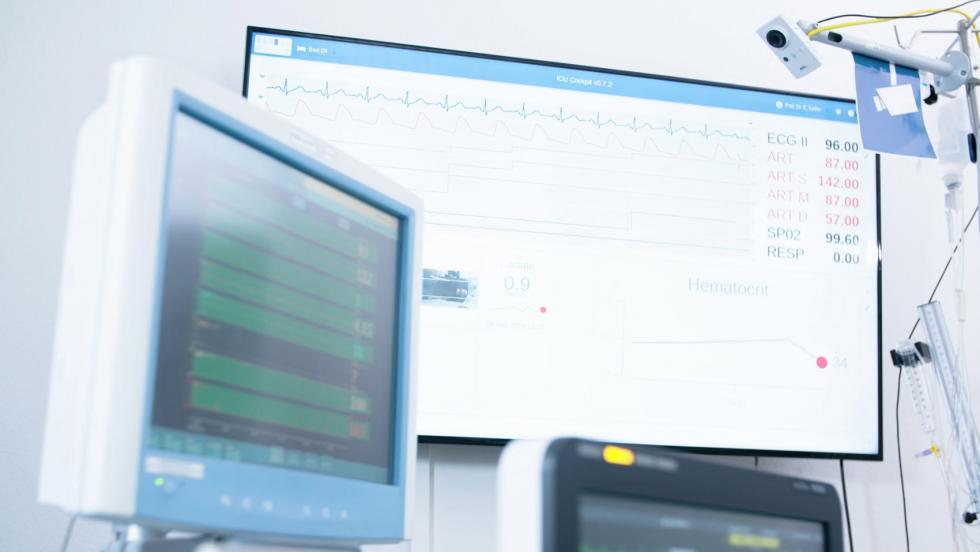
Just a single critical patient treated in an intensive care unit or emergency ward generates up to 100 gigabytes of data every day. The data is generated by the machines that monitor patients, but a great deal of data is also provided by examinations such as computer and magnetic resonance tomography scans of the brain, laboratory results, and biosensors. All too often, it is simply not possible to use this overload of information for the timely identification of risk constellations and for rapid decision-making.
Fewer false alarms – improved patient safety
Conventional monitoring systems trigger some 700 alarms per patient and day, i.e. one alarm approximately every two minutes. A considerable proportion of these are false alarms. If the number of false alarms could be substantially decreased, the quantity of data would be greatly reduced, which would make it easier to identify critical situations and thus increase patient safety. The neurosurgical intensive care unit at the University Hospital Zurich, ETH Zurich, and IBM Research are currently working on this within the framework of the “ICU-Cockpit” project. Project manager Emanuela Keller outlines the long-term goal: “With this project, we want to initiate a fundamental development in emergency and intensive care medicine – and thus significantly improve the everyday working methods in hospitals.”
Early warning of critical events
The project was able to systematically collect data from several sources on more than 400 patients and also used video footage. All the data was anonymized prior to further processing. Intensive care patients are very vulnerable in a number of respects, which makes their data particularly sensitive. Based on this data, the researchers developed procedures for three applications: firstly, for filtering out false alarms, secondly, for the early detection of epileptic seizures, and thirdly, for the early detection of secondary brain damage.
The latter two procedures are designed to identify risk constellations and warn of imminent critical events, in the sense of a prognosis. This allows interventions to be made at an earlier stage, which improves the quality of treatment.
Nowadays, therapeutic decisions are often made empirically, based on the experience and knowledge of the experts in charge of the treatment. It would be desirable to back up these decisions with data analyses that are available in real time, as well as the latest medical knowledge from other sources, for example harmonized global databases. The project demonstrates how this can be achieved.
Visual presentation – automatic risk identification
In the next phase, the procedures are to be tested using additional datasets and then directly implemented within the framework of a study in the daily clinical routine at the Zurich University Hospital. The results of the data analysis are to be displayed visually in the ICU in order to allow risk constellations to be identified automatically. The collaboration with IBM Research, which uses video surveillance to detect epileptic seizures and other neurological disorders, will also be continued. From the researchers’ point of view, these procedures based on video recordings also open up possibilities to improve the monitoring of stroke patients who suffer from paralysis.