SHORT NEWS
Deep learning system explores the inside of materials - from the outside
MIT researchers have developed a new method to find out what is going on inside materials. To do this, they need data from the material's surface alone.
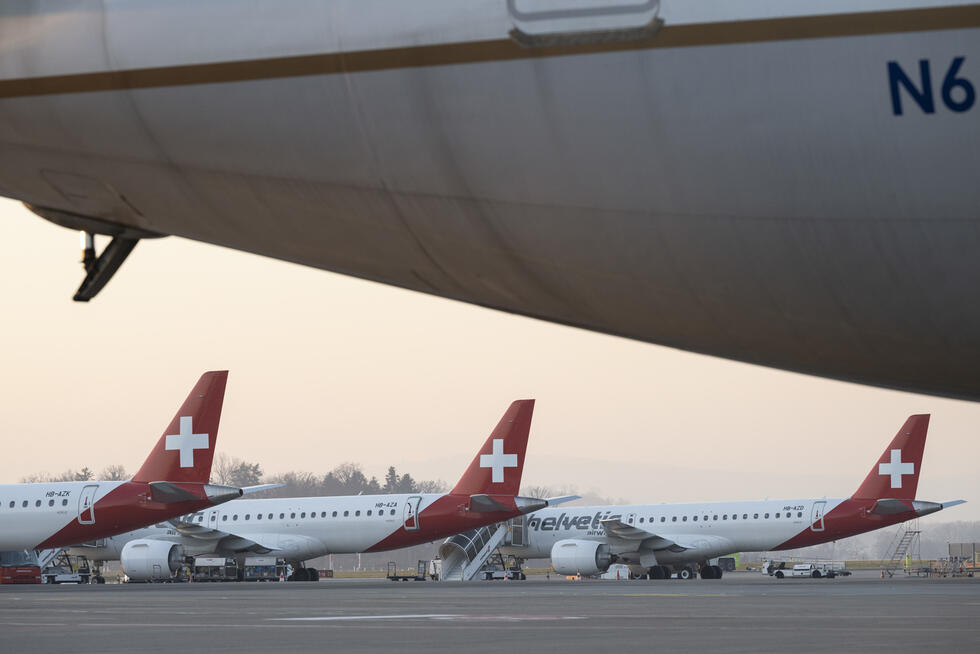
The team used a type of machine learning called deep learning to compare a large set of simulated data about the external force fields of materials with the corresponding internal structure. From this, they developed a system that can make reliable predictions about the interior based on the surface data.
"It's a common problem in engineering: If you have a piece of material - maybe a car door or a part of an aeroplane - and you want to know what's inside, you can measure the strains on the surface by taking pictures and calculating how much deformation you have. But you can't really look inside the material," explains Markus Bühler, professor of civil and environmental engineering at MIT. "You can only do that by cutting it up and then looking inside to see if there is any damage."
Existing methods are more cumbersome
It is also possible to use X-rays and other techniques, but these tend to be expensive and require bulky equipment, he says. "So we basically asked ourselves the question: Can we develop an AI algorithm that looks at what's going on at the surface and then tries to figure out what's going on inside?" This internal information could include damage, cracks or stresses in the material, or details of the internal microstructure.
The same kind of questions can apply to biological tissue, Buehler adds. "Is there disease, some kind of growth or changes in the tissue there?" The goal was to develop a system that could answer these kinds of questions in a completely non-invasive way.
Possible even with mixed materials
In the technique they developed, an AI model was trained using large amounts of data on surface measurements and their associated internal properties. Not only uniform materials were considered, but also combinations of different materials.
As an example of the application of this methodology, Buehler points out that aircraft today are often examined by testing a few representative areas using expensive methods such as X-rays because it would be impractical to test the entire aircraft. "This is a different approach where you can collect data and make predictions in a much less costly way," says Bühler. "Based on that, you can then decide where to look and maybe use more expensive equipment to test."