ANNA - the black box that saves lives
Where reality ends, Gerd Reis’ “Augmented Vision” begins: This is the name of the department at the German Research Center for Artificial Intelligence, where the computer scientist teaches machines not just to see – but also to understand.
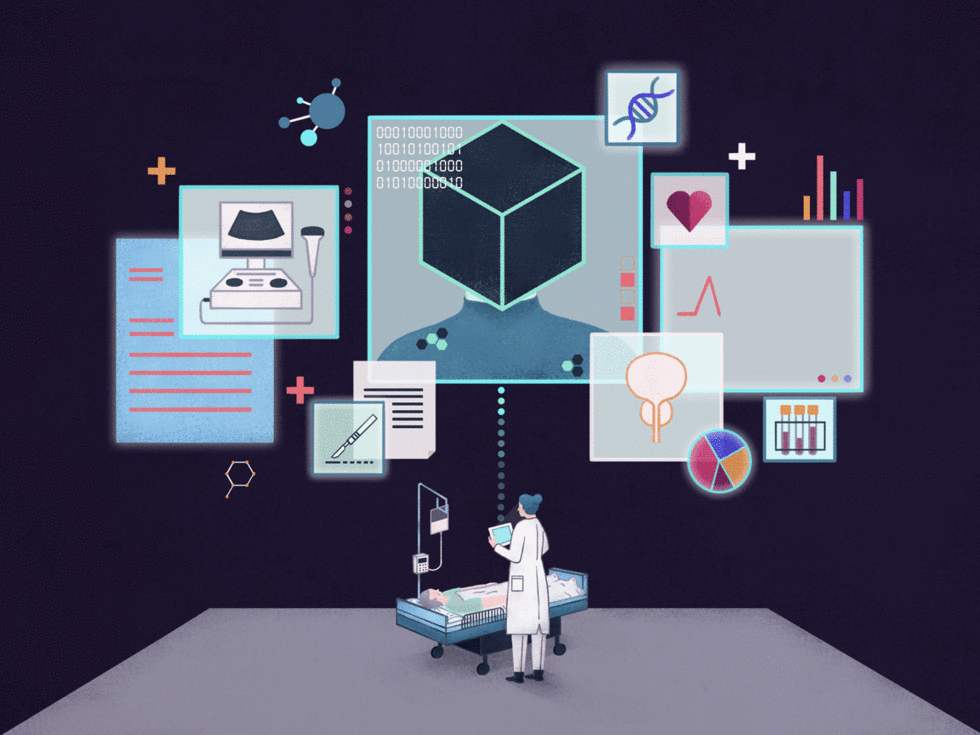
Mr. Reis, your primary field of research is “computer vision”. What is that about?
It is about computers learning to understand what they see in images. Whereby one must be careful with concepts that really apply to humans, such as understanding, seeing, or intelligence. Certainly, a neural network (NN) can achieve things that come close to human cognition. But they are nevertheless entirely different. A child needs just a single encounter to understand what a cat is. NNs require countless training sessions and thousands of cat images to be able to reliably recognize cats. However, at the end of the day, they can do it equally as well as humans, sometimes even better. And NNs are versatile. Regardless of whether their task is to recognize cats, cancer tissue, or road signs. My field of research is so exciting because it is the prerequisite for many promising technologies of the future: autonomous driving, medical diagnostic procedures, or robot vision.
You are also very familiar with one these technologies: You spent many years researching medical diagnostic procedures and trained an NN in this field. It, or rather she, is called “ANNAcTRUS”. ANNA stands for “Artificial Neural Network Analysis”.
Precisely. ANNA is an artificial intelligence that helps diagnose prostate cancer.
How exactly does she work?
ANNA searches for patterns in ultrasound images (TRUS) that are indicative of cancer. We trained her using scans that show positive diagnoses. This taught her what cancer looks like. Or more accurately: Which patterns in the images are indicative of cancer. But we have no idea of precisely how she does it. She is, like most NNs, a black box.
ANNA is already performing more reliably than her human counterparts
You know the solution, but not how it was reached. Isn’t it unethical to entrust people’s health to a black box that you cannot look inside?
It would be unethical to blindly rely on a machine. It is still the medical professionals who are essentially and ultimately liable. Consequently, ANNA does not make any decisions. She simply highlights suspicious areas in the images.
So she acts as an assistant in the everyday routine in clinics.
Precisely. Ideally, the physicians only have to confirm ANNA’s proposals and perform targeted biopsies, i.e. take tissue samples from the highlighted areas. The responsibility for treatment always lies with humans. The results provided by NNs should never be taken as the ultimate truth. We cannot leave a final decision to a bunch of numbers.
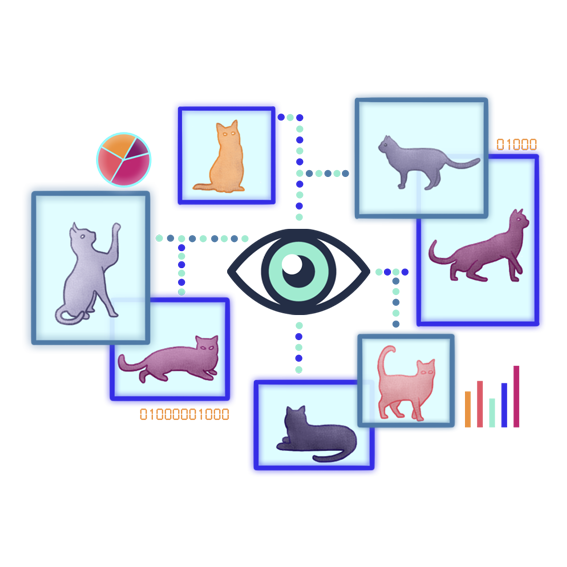
Not even if the NN solves the tasks more effectively and faster than humans?
In my opinion, NNs and AI should be used as tools. ANNA performs more reliably than many urologists and delivers consistently high quality. In addition, she is very fast and thus saves valuable time in everyday clinical work. I view ANNA as a support for people, not as their competitor. ANNA’s work frees up time – for example for face-to-face interaction with patients.
Why is ANNA better than many physicians?
To answer this I must first give you some background information: In the event of prostate cancer, the PSA level – the prostate specific antigen – is usually elevated. However, an elevated PSA level is only an indication of cancer. A tissue sample must be analyzed to provide definitive diagnosis and treatment. But locating prostate cancer is difficult. This is why physicians perform biopsies. To date, determining where exactly this biopsy should be conducted was generally based on the physician’s experience. Now, ANNA uses its examination of the images to propose areas where a biopsy is most likely to yield results. She recognizes patterns that the human eye overlooks. This is because human vision functions by applying edge-enhancement, which results in the emergence of virtual patterns and the blurring of real ones. Biopsies based on ANNA’s proposals have a 40 percent higher hit rate with half the number of required excisions. This reduces the stress on patients and improves patient care.
In future, digitalized medicine will be personalized
Does this also apply to specialists with years of experience?
We compared ANNA to “average medical professionals”. So far, experts who have amassed a great deal of experience during their career are hardly ever outperformed by AI. But such experts are rare. Physicians are people, and it takes a long time to build up the necessary experience.
What makes it so difficult to diagnose cancer?
Nowadays, the images used for cancer examinations are in high resolution and multimodal. This means they originate from computer tomography, magnetic resonance imaging, and ultrasound. Physicians then have to merge and evaluate the different images and forms of presentation in their minds. A cancer diagnosis requires tremendous mental effort.
AI systems, such as ANNA, are increasingly being used in diagnostics. In a recent medical study, a computer program diagnosed kidney failure three days before it actually occurred. Another AI system identified a heart rhythm disorder – in spite of an inconspicuous echocardiogram.
Both sound plausible to me. These are qualities that could soon become commonplace thanks to AI technology. Above all, the fields of “prognosis” and “personalized precision medicine” could take an enormous step forward.
Please elaborate on this.
When AI systems sift through large volumes of medical data, they could, for example, detect connections between genetic dispositions, dietary habits, work habits, and illnesses. Based on this knowledge, AI could give people specific advice for a healthier lifestyle and thus actively prevent diseases from even developing. This is in contrast to our current approach of treating diseases only after they occur.
This is the field of application you already mentioned: prognosis. What about therapy?
AI systems that are trained using data from treatment histories could improve therapies: For example by predicting how a given treatment would affect a patient in their current condition. Or by calculating the optimal dosage of a drug for a patient based on their state of health. Therapies would become more efficient and targeted, and physicians would be able to cut back on the prescription of unnecessary drugs.
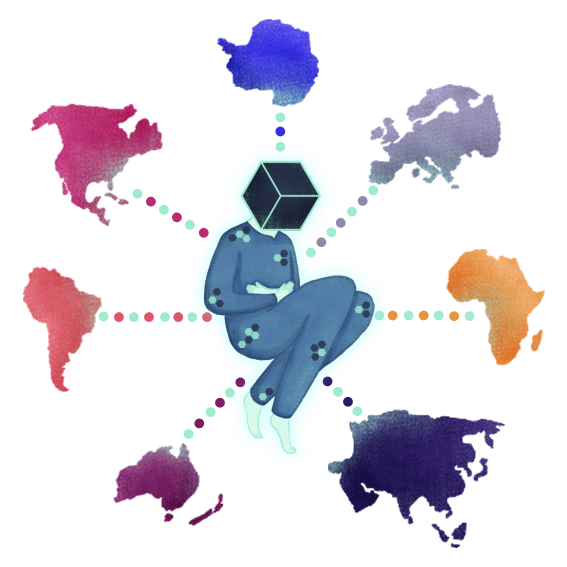
The requirement: a package insert for neural networks
But for this we need medical data on a large scale.
And not just that. The training data must be representative and above all sound. And the results must be widely validated. Otherwise, we will experience a rerun of “Watson”. Watson is an AI system created by the technology company IBM. Based on extensive training data, Watson made therapy recommendations for patients in North America. And its recommendations were good. Watson functioned well in the daily clinical environment. However, when Watson was subsequently tested in Europe, its therapy recommendations were usually off the mark. Most likely because it was trained exclusively using American health data, and the therapy methods used on the two continents are quite different.
So AI does not work universally – what implications does this have in terms of practical application?
AI systems usually only function for the domain from which the data with which they were trained originates. In the case of prostate cancer, for example, studies indicate significant ethnic differences.
So you are advocating a kind of patient information leaflet for NNs?
It should be clear in which data domain the NN was trained. Reliable answers can only be expected within this domain. An NN that has been trained for prostate cancer definitely cannot be used to diagnose breast cancer. Similarly, if an NN has been trained with data from one ethnic group, the utmost caution should be exercised when applying it to another group.
What about non-specific attributes: Is it generally advisable to train NNs with as much data as possible?
Not necessarily. Breast cancer, for example, occurs primarily in women. Very rarely, however, it also affects men. If the attribute “gender” were to play a role in the training of the NN, it could learn that men virtually never suffer from breast cancer. “Male” could then become an exclusion criterion for breast cancer. Attributes that falsify the result have to be filtered out prior to training or weighted appropriately during training.
Otherwise, the NN would discriminate against patients on the basis of certain attributes.
Precisely – in the past we developed an application for the clinical picture of autism. However, it completely failed with black patients. The reason was that the NN had been trained using YouTube videos. The vast majority of the data was obtained from white people. The NN was not able to detect any patterns in the faces of black people.
The German government’s Anti-Discrimination Office recently issued a warning against using skewed datasets in the development of NNs. But how can one obtain comprehensive training data in the medical field that can be used as the basis for the development of better and safer AI systems?
This is difficult. From a technical point of view, I advocate a European center for medical data, where essentially all data is entered anonymously and which NNs could then use to learn. It would be desirable for data from all around the globe to be collected in one place. With the aid of appropriate data curation, this would enable non-restrictive, non-discriminatory NNs to be developed. Federated learning could be an alternative, but research in this field is still in the early stages.
And then what would the future hold for AI in the medical field?
Today NNs see and recognize patterns in images and data. The next big step will be the evaluation of what they see. This will be followed by the prognosis of diseases and disease progression. The next step will be the launch of mobile applications on the mass market: Simple programs that allow first aid or rapid diagnosis using smartphones. Telemedicine will also continue to progress so that it will become possible to speak to a virtual doctor via a video call at any time and from anywhere.
Written by:
Illustration: Liebana Goñi